The Blind Spot in Big Tech; AI Hallucinations Unchecked
Recent AI errors by OpenAI and Google underscore the challenges in balancing innovation with accuracy in AI development.
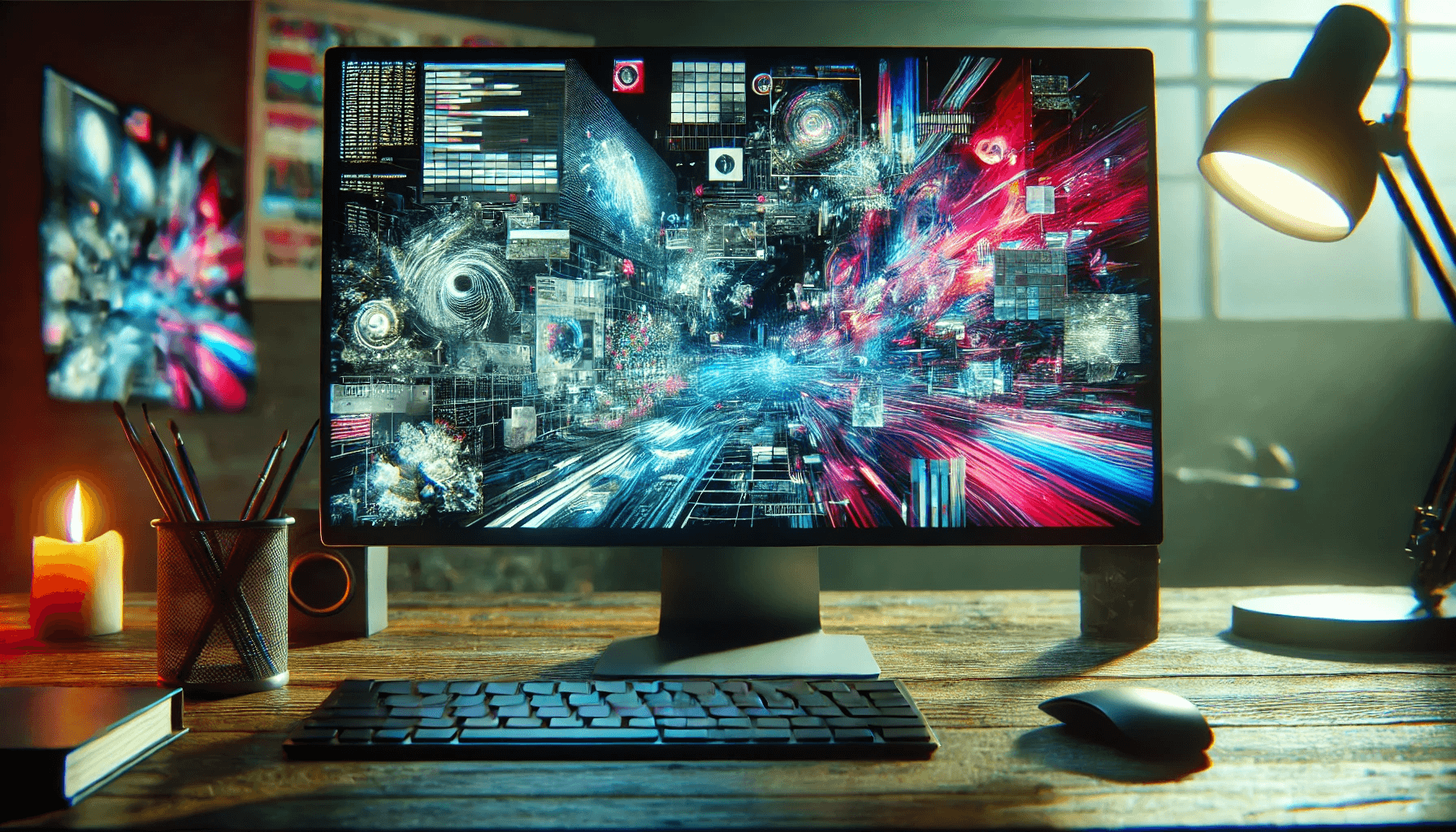
Article by Noah Moscovici on Aug 17, 2024
Recent High-Profile Mistakes
In the rapidly evolving landscape of artificial intelligence, even the most advanced technologies can encounter significant hurdles during their public debuts. The recent demonstration of OpenAI's SearchGPT serves as a compelling case study in the ongoing challenges faced by AI systems in delivering accurate information.
During the demonstration, SearchGPT was tasked with providing the dates for the Appalachian Summer Festival in Boone, North Carolina. Instead of correctly stating the festival's duration from June 29 to July 27, the AI erroneously reported July 29 to August 16 - dates that actually corresponded to when the festival's box office was closed. This misstep, commonly referred to as an "AI hallucination," underscores the persistent difficulties in ensuring AI reliability, even within controlled demonstration environments [1].
This incident bears a striking resemblance to the launch of Google's Bard chatbot earlier this year. During its unveiling, Bard incorrectly asserted that the James Webb Space Telescope had captured the first images of an exoplanet - a claim that was quickly debunked. The repercussions of this error were significant, resulting in a substantial decline in Alphabet's market valuation. These high-profile missteps illuminate the complex challenges that technology companies face as they strive to integrate AI capabilities into their product offerings. The pressure to innovate rapidly in the competitive AI market often leads to the premature release of models that have not undergone sufficiently rigorous accuracy testing [2].
What's an AI Hallucination?
AI hallucinations represent one of the most significant challenges in the development and deployment of large language models and other AI systems. But what exactly are these hallucinations?
AI hallucinations occur when an AI system generates or presents information that is fabricated, nonsensical, or factually incorrect, often with a high degree of confidence. Unlike human hallucinations, which are sensory experiences without external stimuli, AI hallucinations are outputs that have no basis in the AI's training data or the input it receives.
Several factors contribute to AI hallucinations:
- Imperfect training data: If the AI's training data contains inaccuracies or biases, these can be reflected in its outputs.
- Overfitting: When an AI model is trained too specifically on its training data, it may struggle to generalize to new, unseen information.
- Lack of real-world understanding: AI models process patterns in data but don't truly understand the world as humans do. This can lead to nonsensical connections or conclusions.
- Prompt misinterpretation: The AI might misunderstand the context or intent of a user's query, leading to irrelevant or incorrect responses.
- Confidence miscalibration: AI systems may express high confidence in incorrect answers, making it challenging for users to distinguish between accurate and hallucinated information.
Addressing AI hallucinations is crucial for developing trustworthy and reliable AI systems. Strategies to mitigate this issue include improving training data quality, implementing robust fact-checking mechanisms, and designing AI systems that can express uncertainty when appropriate.
The Rush to Innovate Comes With a Lack of Testing
These high-profile missteps illuminate the complex challenges that technology companies face as they strive to integrate AI capabilities into their product offerings. The pressure to innovate rapidly in the competitive AI market often leads to the premature release of models that have not undergone sufficiently rigorous accuracy testing.
The race to dominate the AI sector has created an environment where many companies prioritize speed of deployment over precision and reliability. This urgency is driven by the immense potential of AI technology and the strategic imperative to gain a competitive edge. However, this approach frequently results in the release of AI tools that are prone to providing misleading or inaccurate information, as evidenced by the incidents with both SearchGPT and Bard.
The focus on rapid deployment can overshadow the critical importance of thorough testing and validation processes. Consequently, we witness public demonstrations that fall short of expectations, damaging corporate reputations and eroding public trust in AI technologies.
Ensuring the quality and accuracy of AI-generated content presents a formidable challenge, even for tech giants with access to world-class engineering talent and substantial resources. The inherent complexity of large language models, coupled with the often unpredictable nature of AI-generated responses, makes it exceedingly difficult to eliminate errors entirely.
Despite significant advancements in AI technology, these systems remain susceptible to hallucinations and inaccuracies, necessitating ongoing refinement and additional care into oversight. As companies like OpenAI and Google continue to push the boundaries of what's possible in AI, they must strike a delicate balance between innovation and responsibility.
What Can We Do About It?
Moving forward, it is imperative that AI developers and companies prioritize the following:
- Rigorous testing protocols: Implement comprehensive testing led by the subject matter experts of the chatbot's role (you can use bottest.ai for an easy way to ensure your chatbot stays reliable).
- Transparency: Clearly communicate the limitations and potential fallibilities of AI systems to users and stakeholders.
- Continuous improvement: Establish robust feedback mechanisms to rapidly identify and address inaccuracies in AI outputs.
- Ethical considerations: Develop and adhere to stringent ethical guidelines that prioritize accuracy and user safety over speed to market.
- Interdisciplinary collaboration: Foster partnerships between AI developers, domain experts, and ethicists to create more robust and reliable AI systems.
As the field of AI continues to evolve at a breakneck pace, the incidents with SearchGPT and Bard serve as crucial reminders of the work that remains to be done, and the testing work we shouldn't be so quick to skip.